Reputation Polarity Analysis
A look at how the limitations of sentiment analysis for media reputation management can be overcome, and how Hypefactors is pushing the state-of-the-art in this field.
This is the fourth post a series of posts discussing AI techniques for reputation management. Find the previous post in this series here.
In this blog post we will look at how Hypefactors is pushing the-state-of-the-art of AI assisted reputation management by going beyond the limitations of sentiment analysis as discussed in a previous post.
As discussed in the previous post, we have identified several shortcomings of traditional sentiment analysis systems offered by most PR and reputation management platforms in the market today:
- Sentiment analysis systems are fundamentally incapable of identifying statements that impact your reputation positively or negatively without expressing feelings and opinions.
- Many sentiment systems in use today does not take into account mentions of your brand that express sentiment towards something or someone else, and will in many cases imprecisely assign sentiment to the mention overall.
- The performance of many sentiment systems degrade when applied to news or social media data because these sources are linguistically different from the available training data sources.
In response to these shortcomings we have researched and redesigned an alternative approach which we believe will deliver much greater value than what is possible for other PR management products in the market. The core features of this AI system is:
- Ability to predict whether or not a particular statement will impact your brand’s reputation negatively or positively even when the statement expresses no sentiment.
- Ability to distinguish between different targets of reputation polarity within the same text, and assign labels to them independently.
- Ability to assign reputation polarity labels to text sources in 104 different languages
- Trained on our in house curated datasets to provide accurate predictions on the media sources that are relevant to your brand.
Concretely, consider the following statement taken from a news article describing how Grundfos Lifelink is delivering technology that enables clean drinking water in africa:
Most conventional sentiment analysis systems labels the above statement as negative because of the presence of phrases such as fall into disrepair, be shut down and not a viable approach. Imagine that you are a PR manager at Grundfos Lifelink. The label you are provided by a state-of-the-art sentiment analysis system is technically correct, but its not useful for you to understand how the mention impacts the reputation of Grundfos Lifelink since this is the exact opposite of the sentiment analysis label, namely positive.
“There is plenty of water underground in most areas. The challenge is to make a system which is sustainable. There is no point setting up a system to supply people with water if they cannot afford to maintain it. It will simply fall into disrepair and very quickly be shut down. This is not a viable approach,” says Peter Todbjerg Hansen, Managing Director of Grundfos Lifelink.
First and foremost, we are building our own curated datasets for training machine learning systems to label natural language, not with sentiment, but with what is generally known as reputation polarity.
Reputation polarity analysis (RPA) is concerned with labelling text with the expected impact on the reader’s perception of brand reputation in a negative, neutral or positive direction. In contrast to sentiment analysis, RPA captures most of what you care about for the purpose of reputation management.
Challenges of Reputation Polarity Analysis
From an engineering perspective, RPA is a much more difficult problem than sentiment analysis. An RPA system must be able to do everything a sentiment analysis system can do, since the expression of positive and negative feelings and opinions toward a brand is likely to impact the reader’s perception of its reputation. In addition, an AI system for predicting reputation polarity must also be able to recognize events that are generally associated with negative changes in reputation perception such as product recalls, or events associated with positive changes in reputation perception such as charity contributions.
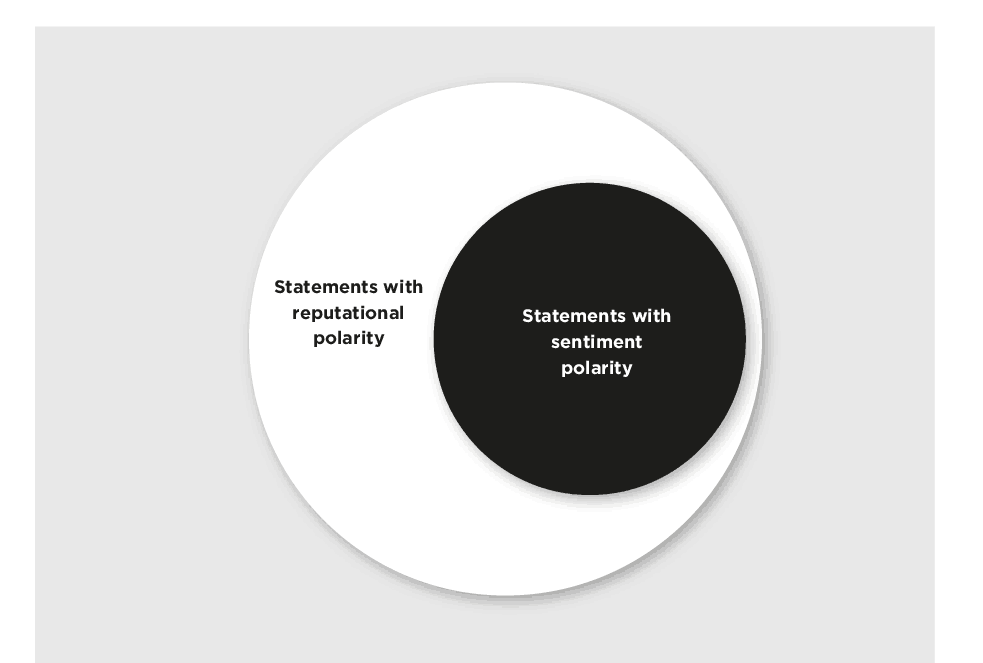
Finally, the impact of certain events on the reputation of your organization can depend on the type of your organization or brand. For example, if you are a tax-funded organization, throwing an extravagant party may influence your reputation negatively. If you are a private company on the other hand, the same event may not impact your reputation or may perhaps even impact it positively. A successful RPA system must learn to make these complicated distinctions as well, which requires a model of facts that are often not immediately expressed in the text itself.
Targeted Reputation Polarity Analysis
In addition to tackling these difficult problems, our AI system is designed to make distinctions about different targets of reputation polarity within the same piece of text. This enables us to provide correct labels for the United Airlines example in the previous section, depending on whether the target is considered to be United Airlines or Donald Trump.
Finally we are using state-of-the-art techniques within a field of machine learning called transfer learning to provide reputation polarity analysis for 104 languages. This allows our customers to get the value of our AI systems even for truly global brands.
The Hypefactors PRA system is designed to label text with precisely this information. We believe that this improvement makes our AI system far more useful than existing solutions.
Conclusion
In this final post in our series about AI techniques for reputation management, we have seen how reputation polarity analysis gives you much more relevant information about the reputation of your brand when compared to the more common approach of sentiment analysis.
We have also discussed how Hypefactors is pushing the state-of-the-art in this field, by building AI systems that can distinguish between several targets of reputation polarity within the same piece of text which improves the accuracy of our solution in many cases.
If you want to know more about how Hypefactors can help you manage your brand’s reputation and public relations, contact us today.